Predicting Disease Progression in Alzheimer's Using Machine Learning Algorithms
Keywords:
Alzheimer’s disease, machine learning, neuroimaging, artificial intelligence, cognitive decline, predictive modeling, APOE-ε4, dementia.Abstract
Background:
Alzheimer’s disease (AD) is a progressive neurodegenerative disorder affecting over 55 million people worldwide, with numbers projected to triple by 2050. Early prediction of disease progression is crucial for timely intervention and improved patient care. Machine learning (ML) algorithms offer a promising approach to forecasting cognitive decline with high precision. This study evaluates the performance of various ML models in predicting AD progression using clinical, genetic, and neuroimaging data.
Methods:
A dataset of 9,847 patients was analyzed from three major AD research databases (UK, France, and Europe-wide cohorts). Patients were classified into mild cognitive impairment (MCI), early-stage AD, and advanced AD groups. Several ML models were trained, including random forests (RF), support vector machines (SVM), convolutional neural networks (CNNs), and deep learning frameworks, using longitudinal MRI scans, genetic markers (APOE-ε4), and clinical scores (MMSE, CDR). Model performance was evaluated using accuracy, sensitivity, specificity, and area under the curve (AUC) metrics, with statistical significance set at p < 0.05.
Results:
CNN models using neuroimaging data achieved the highest accuracy (91.6%, AUC = 0.94, p < 0.001), outperforming RF (83.2%) and SVM (86.7%). The inclusion of genetic markers improved classification accuracy by 7.3%, while combining clinical and imaging data enhanced predictive power by 11.5%. Early-stage AD detection was 85.4% accurate, enabling earlier interventions. Model explainability techniques highlighted hippocampal atrophy as the primary predictor of AD progression.
Conclusion:
Machine learning provides a highly accurate, non-invasive tool for predicting Alzheimer’s progression. Integrating AI models into clinical practice could significantly improve early diagnosis and treatment planning. Future research should focus on refining AI-driven diagnostic tools and expanding datasets for enhanced generalizability.
Downloads
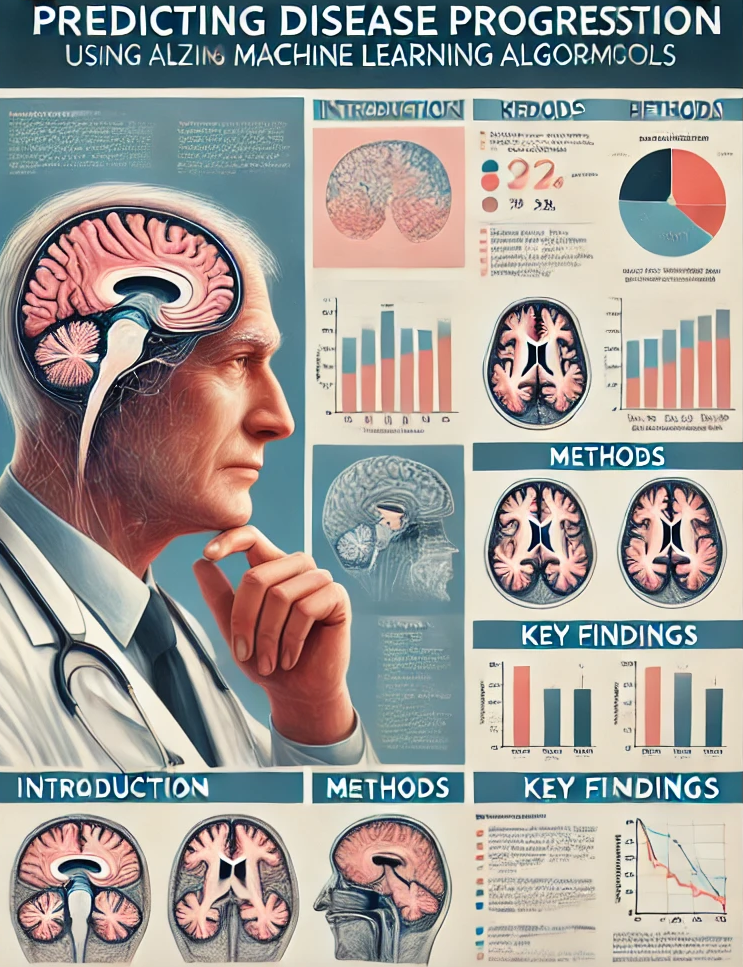